Solution Manual Artificial Intelligence 3rd Russell
Solutions to AIMA (Artificial Intelligence: A Modern Approach). Solutions to Russell and Norvig's Artificial Intelligence: A Modern Approach (AIMA, 3rd edition). Get this from a library! Artificial intelligence: a modern approach, instructor's solutions manual. [Stuart J Russell; Peter Norvig].
This work is protected by local and international copyright laws and is provided solely for the use of instructors in teaching their courses and assessing student learning. Dissemination or sale of any part of this work (including on the World Wide Web) will destroy the integrity of the work and is not permitted. The work and materials from this site should never be made available to students except by instructors using the accompanying text in their classes. All recipients of this work are expected to abide by these restrictions and to honor the intended pedagogical purposes and the needs of other instructors who rely on these materials.
Nontechnical learning material. Provides a simple overview of major concepts, uses a nontechnical language to help increase understanding.
Makes the book accessible to a broader range of students. The Internet as a sample application for intelligent systems — Examples of logical reasoning, planning, and natural language processing using Internet agents. Promotes student interest with interesting, relevant exercises. Increased coverage of material — New or expanded coverage of constraint satisfaction, local search planning methods, multi-agent systems, game theory, statistical natural language processing and uncertain reasoning over time. More detailed descriptions of algorithms for probabilistic inference, fast propositional inference, probabilistic learning approaches including EM, and other topics.
Brings students up to date on the latest technologies, and presents concepts in a more unified manner. Updated and expanded exercises — 30% of the exercises are revised or NEW. More Online Software. Allows many more opportunities for student projects on the web. A unified, agent-based approach to AI — Organizes the material around the task of building intelligent agents. Shows students how the various subfields of AI fit together to build actual, useful programs. Comprehensive, up-to-date coverage — Includes a unified view of the field organized around the rational decision making paradigm.
A flexible format. Makes the text adaptable for varying instructors' preferences.
In-depth coverage of basic and advanced topics. Provides students with a basic understanding of the frontiers of AI without compromising complexity and depth. Pseudo-code versions of the major AI algorithms are presented in a uniform fashion, and Actual Common Lisp and Python implementations of the presented algorithms are available via the Internet. Gives instructors and students a choice of projects; reading and running the code increases understanding. Author Maintained Website. Visit to access text-related Comments and Discussions, AI Resources on the Web, and Online Code Repository, Instructor Resources, and more!
Artificial Intelligence Movie
New To This Edition This edition captures the changes in AI that have taken place since the last edition in 2003. There have been important applications of AI technology, such as the widespread deployment of practical speech recognition, machine translation, autonomous vehicles, and household robotics. There have been algorithmic landmarks, such as the solution of the game of checkers. And there has been a great deal of theoretical progress, particularly in areas such as probabilistic reasoning, machine learning, and computer vision. Most important from the authors' point of view is the continued evolution in how we think about the field, and thus how the book is organized. The major changes are as follows:. More emphasis is placed on partially observable and nondeterministic environments, especially in the nonprobabilistic settings of search and planning.
The concepts of belief state (a set of possible worlds) and state estimation (maintaining the belief state) are introduced in these settings; later in the book, probabilities are added. In addition to discussing the types of environments and types of agents, there is more in more depth coverage of the types of representations that an agent can use. Differences between atomic representations (in which each state of the world is treated as a black box), factored representations (in which a state is a set of attribute/value pairs), and structured representations (in which the world consists of objects and relations between them) are distinguished. Coverage of planning goes into more depth on contingent planning in partially observable environments and includes a new approach to hierarchical planning.
New material on first-order probabilistic models is added, including open-universe models for cases where there is uncertainty as to what objects exist. The introductory machine-learning chapter is completely rewritten, stressing a wider variety of more modern learning algorithms and placing them on a firmer theoretical footing. Expanded coverage of Web search and information extraction, and of techniques for learning from very large data sets. 20% of the citations in this edition are to works published after 2003.
Approximately 20% of the material is brand new. The remaining 80% reflects older work but is largely rewritten to present a more unified picture of the field.
Table of Contents I. Artificial Intelligence 1. Introduction 1.1 What is AI? 1.2 The Foundations of Artificial Intelligence 1.3 The History of Artificial Intelligence 1.4 The State of the Art 1.5 Summary, Bibliographical and Historical Notes, Exercises 2.
Intelligent Agents 2.1 Agents and Environments 2.2 Good Behavior: The Concept of Rationality 2.3 The Nature of Environments 2.4 The Structure of Agents 2.5 Summary, Bibliographical and Historical Notes, Exercises II. Problem-solving 3. Solving Problems by Searching 3.1 Problem-Solving Agents 3.2 Example Problems 3.3 Searching for Solutions 3.4 Uninformed Search Strategies 3.5 Informed (Heuristic) Search Strategies 3.6 Heuristic Functions 3.7 Summary, Bibliographical and Historical Notes, Exercises 4. Beyond Classical Search 4.1 Local Search Algorithms and Optimization Problems 4.2 Local Search in Continuous Spaces 4.3 Searching with Nondeterministic Actions 4.4 Searching with Partial Observations 4.5 Online Search Agents and Unknown Environments 4.6 Summary, Bibliographical and Historical Notes, Exercises 5. Adversarial Search 5.1 Games 5.2 Optimal Decisions in Games 5.3 Alpha—Beta Pruning 5.4 Imperfect Real-Time Decisions 5.5 Stochastic Games 5.6 Partially Observable Games 5.7 State-of-the-Art Game Programs 5.8 Alternative Approaches 5.9 Summary, Bibliographical and Historical Notes, Exercises 6. Constraint Satisfaction Problems 6.1 Defining Constraint Satisfaction Problems 6.2 Constraint Propagation: Inference in CSPs 6.3 Backtracking Search for CSPs 6.4 Local Search for CSPs 6.5 The Structure of Problems 6.6 Summary, Bibliographical and Historical Notes, Exercises III.
Knowledge, Reasoning, and Planning 7. Logical Agents 7.1 Knowledge-Based Agents 7.2 The Wumpus World 7.3 Logic 7.4 Propositional Logic: A Very Simple Logic 7.5 Propositional Theorem Proving 7.6 Effective Propositional Model Checking 7.7 Agents Based on Propositional Logic 7.8 Summary, Bibliographical and Historical Notes, Exercises 8. First-Order Logic 8.1 Representation Revisited 8.2 Syntax and Semantics of First-Order Logic 8.3 Using First-Order Logic 8.4 Knowledge Engineering in First-Order Logic 8.5 Summary, Bibliographical and Historical Notes, Exercises 9. Inference in First-Order Logic 9.1 Propositional vs. First-Order Inference 9.2 Unification and Lifting 9.3 Forward Chaining 9.4 Backward Chaining 9.5 Resolution 9.6 Summary, Bibliographical and Historical Notes, Exercises 10. Classical Planning 10.1 Definition of Classical Planning 10.2 Algorithms for Planning as State-Space Search 10.3 Planning Graphs 10.4 Other Classical Planning Approaches 10.5 Analysis of Planning Approaches 10.6 Summary, Bibliographical and Historical Notes, Exercises 11.
Planning and Acting in the Real World 11.1 Time, Schedules, and Resources 11.2 Hierarchical Planning 11.3 Planning and Acting in Nondeterministic Domains 11.4 Multiagent Planning 11.5 Summary, Bibliographical and Historical Notes, Exercises 12 Knowledge Representation 12.1 Ontological Engineering 12.2 Categories and Objects 12.3 Events 12.4 Mental Events and Mental Objects 12.5 Reasoning Systems for Categories 12.6 Reasoning with Default Information 12.7 The Internet Shopping World 12.8 Summary, Bibliographical and Historical Notes, Exercises IV. Uncertain Knowledge and Reasoning 13. Quantifying Uncertainty 13.1 Acting under Uncertainty 13.2 Basic Probability Notation 13.3 Inference Using Full Joint Distributions 13.4 Independence 13.5 Bayes’ Rule and Its Use 13.6 The Wumpus World Revisited 13.7 Summary, Bibliographical and Historical Notes, Exercises 14. Probabilistic Reasoning 14.1 Representing Knowledge in an Uncertain Domain 14.2 The Semantics of Bayesian Networks 14.3 Efficient Representation of Conditional Distributions 14.4 Exact Inference in Bayesian Networks 14.5 Approximate Inference in Bayesian Networks 14.6 Relational and First-Order Probability Models 14.7 Other Approaches to Uncertain Reasoning 14.8 Summary, Bibliographical and Historical Notes, Exercises 15. Probabilistic Reasoning over Time 15.1 Time and Uncertainty 15.2 Inference in Temporal Models 15.3 Hidden Markov Models 15.4 Kalman Filters 15.5 Dynamic Bayesian Networks 15.6 Keeping Track of Many Objects 15.7 Summary, Bibliographical and Historical Notes, Exercises 16. Making Simple Decisions 16.1 Combining Beliefs and Desires under Uncertainty 16.2 The Basis of Utility Theory 16.3 Utility Functions 16.4 Multiattribute Utility Functions 16.5 Decision Networks 16.6 The Value of Information 16.7 Decision-Theoretic Expert Systems 16.8 Summary, Bibliographical and Historical Notes, Exercises 17.
Making Complex Decisions 17.1 Sequential Decision Problems 17.2 Value Iteration 17.3 Policy Iteration 17.4 Partially Observable MDPs 17.5 Decisions with Multiple Agents: Game Theory 17.6 Mechanism Design 17.7 Summary, Bibliographical and Historical Notes, Exercises V. Learning from Examples 18.1 Forms of Learning 18.2 Supervised Learning 18.3 Learning Decision Trees 18.4 Evaluating and Choosing the Best Hypothesis 18.5 The Theory of Learning 18.6 Regression and Classification with Linear Models 18.7 Artificial Neural Networks 18.8 Nonparametric Models 18.9 Support Vector Machines 18.10 Ensemble Learning 18.11 Practical Machine Learning 18.12 Summary, Bibliographical and Historical Notes, Exercises 19.
Knowledge in Learning 19.1 A Logical Formulation of Learning 19.2 Knowledge in Learning 19.3 Explanation-Based Learning 19.4 Learning Using Relevance Information 19.5 Inductive Logic Programming 19.6 Summary, Bibliographical and Historical Notes, Exercises 20. Learning Probabilistic Models 20.1 Statistical Learning 20.2 Learning with Complete Data 20.3 Learning with Hidden Variables: The EM Algorithm 20.4 Summary, Bibliographical and Historical Notes, Exercises 21. Reinforcement Learning 21.1 Introduction 21.2 Passive Reinforcement Learning 21.3 Active Reinforcement Learning 21.4 Generalization in Reinforcement Learning 21.5 Policy Search 21.6 Applications of Reinforcement Learning 21.7 Summary, Bibliographical and Historical Notes, Exercises VI. Communicating, Perceiving, and Acting 22. Natural Language Processing 22.1 Language Models 22.2 Text Classification 22.3 Information Retrieval 22.4 Information Extraction 22.5 Summary, Bibliographical and Historical Notes, Exercises 23.
Natural Language for Communication 23.1 Phrase Structure Grammars 23.2 Syntactic Analysis (Parsing) 23.3 Augmented Grammars and Semantic Interpretation 23.4 Machine Translation 23.5 Speech Recognition 23.6 Summary, Bibliographical and Historical Notes, Exercises 24. Perception 24.1 Image Formation 24.2 Early Image-Processing Operations 24.3 Object Recognition by Appearance 24.4 Reconstructing the 3D World 24.5 Object Recognition from Structural Information 24.6 Using Vision 24.7 Summary, Bibliographical and Historical Notes, Exercises 25. Robotics 25.1 Introduction 25.2 Robot Hardware 25.3 Robotic Perception 25.4 Planning to Move 25.5 Planning Uncertain Movements 25.6 Moving 25.7 Robotic Software Architectures 25.8 Application Domains 25.9 Summary, Bibliographical and Historical Notes, Exercises VII. Conclusions 26 Philosophical Foundations 26.1 Weak AI: Can Machines Act Intelligently? 26.2 Strong AI: Can Machines Really Think? 26.3 The Ethics and Risks of Developing Artificial Intelligence 26.4 Summary, Bibliographical and Historical Notes, Exercises 27.
AI: The Present and Future 27.1 Agent Components 27.2 Agent Architectures 27.3 Are We Going in the Right Direction? 27.4 What If AI Does Succeed? Appendices A. Mathematical Background A.1 Complexity Analysis and O Notation A.2 Vectors, Matrices, and Linear Algebra A.3 Probability Distributions B. Notes on Languages and Algorithms B.1 Defining Languages with Backus—Naur Form (BNF) B.2 Describing Algorithms with Pseudocode B.3 Online Help Bibliography Index.
Alternative Versions Alternative Versions are designed to give your students more value and flexibility by letting them choose the format of their text, from physical books to ebook versions. Pearson offers special pricing when you choose to package your text with other student resources. If you're interested in creating a cost-saving package for your students, see the. Artificial Intelligence: A Modern Approach, eBook, Global Edition, 3/E Russell & Norvig ISBN-10:. ISBN-13: 971 ©2017. Portable Documents.
Instock Net price: £36.66? Pearson Learning Solutions Nobody is smarter than you when it comes to reaching your students. You know how to convey knowledge in a way that is relevant and relatable to your class. It's the reason you always get the best out of them.
And when it comes to planning your curriculum, you know which course materials express the information in the way that’s most consistent with your teaching. That’s why we give you the option to personalise your course material using just the Pearson content you select.
Take only the most applicable parts of your favourite materials and combine them in any order you want. You can even integrate your own material if you wish.

It's fast, it's easy and fewer course materials help minimise costs for your students. For more information: “Creating a personalised resource was a constructive and positive course development for me, as everything is now integrated, aligned and consistent.” — John Sanders, School of Management and Languages, Heriot-Watt University, UK'. Personalised Content Solutions Explore our range of textbook content across the disciplines and see how you can create your own textbook or eBook. Custom textbooks and eBooks Pick and choose content from one or more texts plus carefully-selected third-party content, and combine it into a bespoke book, unique to your course. You can also include skills content, your own material and brand it to your course and your institution.
Read about Durham University's experience of creating a bespoke course eBook for their engineering students. Personalised Digital Solutions Pearson Learning Solutions will partner with you to create a completely bespoke technology solution to your course's specific requirements and needs. Develop websites just for your course, acting as a bespoke 'one-stop shop' for you and your students to access eBooks, MyLab or Mastering courses, videos and your own original material. Include highly engaging bespoke games, animations and simulations to aid students' understanding, promote active learning and accommodate their differing learning styles. Customise existing Pearson eLearning content to match the specific needs of your course. Simply share your course goals with our world-class experts, and they will offer you a selection of outstanding, up-to-the-minute solutions.
For more information.
Tony Garner/BAE BAE Systems' Taranis drone has autonomous elements, but relies on humans for combat decisions. Stuart Russell: Sabine Hauert: Russ Altman: Manuela Veloso: Stuart Russell: Take a stand on AI weapons Professor of computer science, University of California, Berkeley The artificial intelligence (AI) and robotics communities face an important ethical decision: whether to support or oppose the development of lethal autonomous weapons systems (LAWS).
Technologies have reached a point at which the deployment of such systems is — practically if not legally — feasible within years, not decades. The stakes are high: LAWS have been described as the third revolution in warfare, after gunpowder and nuclear arms. Autonomous weapons systems select and engage targets without human intervention; they become lethal when those targets include humans.
LAWS might include, for example, armed quadcopters that can search for and eliminate enemy combatants in a city, but do not include cruise missiles or remotely piloted drones for which humans make all targeting decisions. Existing AI and robotics components can provide physical platforms, perception, motor control, navigation, mapping, tactical decision-making and long-term planning. They just need to be combined.
For example, the technology already demonstrated for self-driving cars, together with the human-like tactical control learned by DeepMind's DQN system, could support urban search-and-destroy missions. Two US Defense Advanced Research Projects Agency (DARPA) programmes foreshadow planned uses of LAWS: Fast Lightweight Autonomy (FLA) and Collaborative Operations in Denied Environment (CODE). The FLA project will program tiny rotorcraft to manoeuvre unaided at high speed in urban areas and inside buildings. CODE aims to develop teams of autonomous aerial vehicles carrying out “all steps of a strike mission — find, fix, track, target, engage, assess” in situations in which enemy signal-jamming makes communication with a human commander impossible.
Other countries may be pursuing clandestine programmes with similar goals. International humanitarian law — which governs attacks on humans in times of war — has no specific provisions for such autonomy, but may still be applicable. The 1949 Geneva Convention on humane conduct in war requires any attack to satisfy three criteria: military necessity; discrimination between combatants and non-combatants; and proportionality between the value of the military objective and the potential for collateral damage. (Also relevant is the Martens Clause, added in 1977, which bans weapons that violate the “principles of humanity and the dictates of public conscience.”) These are subjective judgments that are difficult or impossible for current AI systems to satisfy. The United Nations has held a series of meetings on LAWS under the auspices of the Convention on Certain Conventional Weapons (CCW) in Geneva, Switzerland.
Within a few years, the process could result in an international treaty limiting or banning autonomous weapons, as happened with blinding laser weapons in 1995; or it could leave in place the status quo, leading inevitably to an arms race. As an AI specialist, I was asked to provide expert testimony for the third major meeting under the CCW, held in April, and heard the statements made by nations and non-governmental organizations. Several countries pressed for an immediate ban. Germany said that it “will not accept that the decision over life and death is taken solely by an autonomous system”; Japan stated that it “has no plan to develop robots with humans out of the loop, which may be capable of committing murder” (see ). The United States, the United Kingdom and Israel — the three countries leading the development of LAWS technology — suggested that a treaty is unnecessary because they already have internal weapons review processes that ensure compliance with international law.
Almost all states who are party to the CCW agree with the need for 'meaningful human control' over the targeting and engagement decisions made by robotic weapons. Unfortunately, the meaning of 'meaningful' is still to be determined.
The debate has many facets. Some argue that the superior effectiveness and selectivity of autonomous weapons can minimize civilian casualties by targeting only combatants. Others insist that LAWS will lower the threshold for going to war by making it possible to attack an enemy while incurring no immediate risk; or that they will enable terrorists and non-state-aligned combatants to inflict catastrophic damage on civilian populations. LAWS could violate fundamental principles of human dignity by allowing machines to choose whom to kill — for example, they might be tasked to eliminate anyone exhibiting 'threatening behaviour'. The potential for LAWS technologies to bleed over into peacetime policing functions is evident to human-rights organizations and drone manufacturers. In my view, the overriding concern should be the probable endpoint of this technological trajectory. The capabilities of autonomous weapons will be limited more by the laws of physics — for example, by constraints on range, speed and payload — than by any deficiencies in the AI systems that control them.
For instance, as flying robots become smaller, their manoeuvrability increases and their ability to be targeted decreases. They have a shorter range, yet they must be large enough to carry a lethal payload — perhaps a one-gram shaped charge to puncture the human cranium. Despite the limits imposed by physics, one can expect platforms deployed in the millions, the agility and lethality of which will leave humans utterly defenceless. This is not a desirable future. The AI and robotics science communities, represented by their professional societies, are obliged to take a position, just as physicists have done on the use of nuclear weapons, chemists on the use of chemical agents and biologists on the use of disease agents in warfare.
Debates should be organized at scientific meetings; arguments studied by ethics committees; position papers written for society publications; and votes taken by society members. Doing nothing is a vote in favour of continued development and deployment. Sabine Hauert: Shape the debate, don't shy from it Lecturer in robotics, University of Bristol Irked by hyped headlines that foster fear or overinflate expectations of robotics and artificial intelligence (AI), some researchers have stopped communicating with the media or the public altogether. But we must not disengage. The public includes taxpayers, policy-makers, investors and those who could benefit from the technology. They hear a mostly one-sided discussion that leaves them worried that robots will take their jobs, fearful that AI poses an existential threat, and wondering whether laws should be passed to keep hypothetical technology 'under control'.
My colleagues and I spend dinner parties explaining that we are not evil but instead have been working for years to develop systems that could help the elderly, improve health care, make jobs safer and more efficient, and allow us to explore space or beneath the oceans. Joseph Bibby/NASA NASA's Robonaut 2 could be used in medicine and industry as well as space-station construction. Experts need to become the messengers. Through social media, researchers have a public platform that they should use to drive a balanced discussion. We can talk about the latest developments and limitations, provide the big picture and demystify the technology.
Pramac gbl 42 manual. I have used social media to crowd-source designs for swarming nanobots to treat cancer. And I found my first PhD student through his nanomedicine blog. The AI and robotics community needs thought leaders who can engage with prominent commentators such as physicist Stephen Hawking and entrepreneur–inventor Elon Musk and set the agenda at international meetings such as the World Economic Forum in Davos, Switzerland. Public engagement also drives funding. Crowdfunding for JIBO, a personal robot for the home developed by Cynthia Breazeal, at the Massachusetts Institute of Technology (MIT) in Cambridge, raised more than US$2.2 million.
There are hurdles. First, many researchers have never tweeted, blogged or made a YouTube video.
Second, outreach is 'yet another thing to do', and time is limited. Third, it can take years to build a social-media following that makes the effort worthwhile. And fourth, engagement work is rarely valued in research assessments, or regarded seriously by tenure committees. Training, support and incentives are needed. All three are provided by Robohub.org, of which I am co-founder and president.
Launched in 2012, Robohub is dedicated to connecting the robotics community to the public. We provide crash courses in science communication at major AI and robotics conferences on how to use social media efficiently and effectively.
We invite professional science communicators and journalists to help researchers to prepare an article about their work. The communicators explain how to shape messages to make them clear and concise and avoid pitfalls, but we make sure the researcher drives the story and controls the end result. We also bring video cameras and ask researchers who are presenting at conferences to pitch their work to the public in five minutes. The results are uploaded to YouTube.
We have built a portal for disseminating blogs and tweets, amplifying their reach to tens of thousands of followers. “Through social media, researchers have a public platform that they should use to drive a balanced discussion.” I can list all the benefits of science communication, but the incentive must come from funding agencies and institutes. Citations cannot be the only measure of success for grants and academic progression; we must also value shares, views, comments or likes. MIT robotics researcher Rodney Brooks's classic 1986 paper on the 'subsumption architecture', a bio-inspired way to program robots to react to their environment, gathered nearly 10,000 citations in 30 years.
A video of Sawyer, a robot developed by Brooks's company Rethink Robotics, received more than 60,000 views in one month (see ). Which has had more impact on today's public discourse?
Governments, research institutes, business-development agencies, and research and industry associations do welcome and fund outreach and science-communication efforts. But each project develops its own strategy, resulting in pockets of communication that have little reach. In my view, AI and robotics stakeholders worldwide should pool a small portion of their budgets (say 0.1%) to bring together these disjointed communications and enable the field to speak more loudly. Special-interest groups, such as the Small Unmanned Aerial Vehicles Coalition that is promoting a US market for commercial drones, are pushing the interests of major corporations to regulators. There are few concerted efforts to promote robotics and AI research in the public sphere.
This balance is badly needed. A common communications strategy will empower a new generation of roboticists that is deeply connected to the public and able to hold its own in discussions.
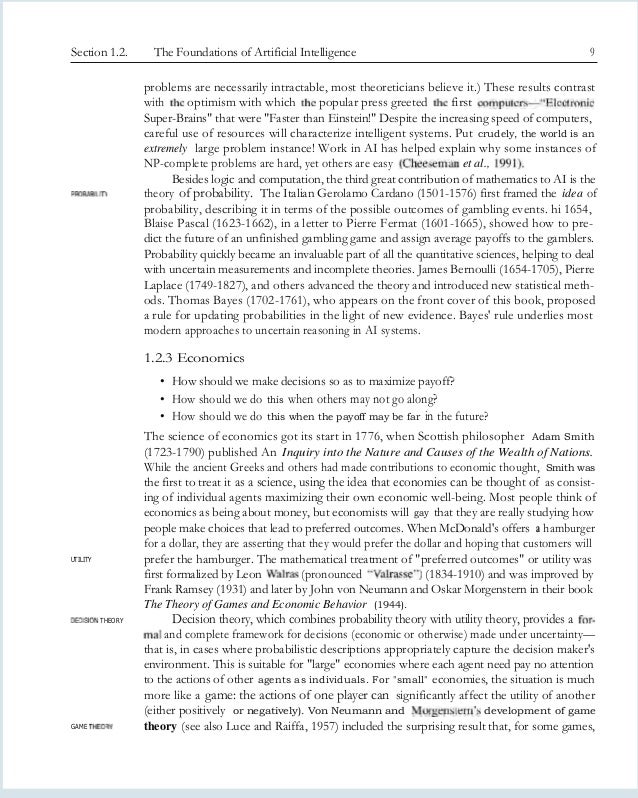
This is essential if we are to counter media hype and prevent misconceptions from driving perception, policy and funding decisions. Russ Altman: Distribute AI benefits fairly Professor of bioengineering, genetics, medicine and computer science, Stanford University Artificial intelligence (AI) has astounding potential to accelerate scientific discovery in biology and medicine, and to transform health care. AI systems promise to help make sense of several new types of data: measurements from the 'omics' such as genomics, proteomics and metabolomics; electronic health records; and digital-sensor monitoring of health signs. Clustering analyses can define new syndromes — separating diseases that were thought to be the same and unifying others that have the same underlying defects. Pattern-recognition technologies may match disease states to optimal treatments.
For example, my colleagues and I are identifying groups of patients who are likely to respond to drugs that regulate the immune system on the basis of clinical and transcriptomic features. In consultations, physicians might be able to display data from a 'virtual cohort' of patients who are similar to the one sitting next to them and use it to weigh up diagnoses, treatment options and the statistics of outcomes. They could make medical decisions interactively with such a system or use simulations to predict outcomes on the basis of the patient's data and that of the virtual cohort. “AI technologies could exacerbate existing health-care disparities and create new ones.” I have two concerns. First, AI technologies could exacerbate existing health-care disparities and create new ones unless they are implemented in a way that allows all patients to benefit. In the United States, for example, people without jobs experience diverse levels of care.
A two-tiered system in which only special groups or those who can pay — and not the poor — receive the benefits of advanced decision-making systems would be unjust and unfair. It is the joint responsibility of the government and those who develop the technology and support the research to ensure that AI technologies are distributed equally. Second, I worry about clinicians' ability to understand and explain the output of high-performance AI systems. Most health-care providers will not accept a complex treatment recommendation from a decision-support system without a clear description of how and why it was reached. Unfortunately, the better the AI system, the harder it often is to explain.
The features that contribute to probability-based assessments such as Bayesian analyses are straightforward to present; deep-learning networks, less so. AI researchers who create the infrastructure and technical capabilities for these systems need to engage doctors, nurses, patients and others to understand how they will be used, and used fairly. Manuela Veloso: Embrace a robot–human world Professor of computer science, Carnegie Mellon University Humans seamlessly integrate perception, cognition and action.
We use our sensors to assess the state of the world, our brains to think and choose actions to achieve objectives, and our bodies to execute those actions. My research team is trying to build robots that are capable of doing the same — with artificial sensors (cameras, microphones and scanners), algorithms and actuators, which control the mechanisms. But autonomous robots and humans differ greatly in their abilities. Robots may always have perceptual, cognitive and actuation limitations. They might not be able to fully perceive a scene, recognize or manipulate any object, understand all spoken or written language, or navigate in any terrain. I think that robots will complement humans, not supplant them. But robots need to know when to ask for help and how to express their inner workings.
Corbis Kirobo, Japan's first robot astronaut, was deployed to the International Space Station in 2013. To learn more about how robots and humans work together, for the past three years we have shared our laboratory and buildings with four collaborative robots, or CoBots, which we developed. The robots look a bit like mechanical lecterns. They have omnidirectional wheels that enable them to steer smoothly around obstacles; camera and lidar systems to provide depth vision; computers for processing; screens for communication; and a basket to carry things in.
Early on, we realized how challenging real environments are for robots. The CoBots cannot recognize every object they encounter; lacking arms or hands they struggle to open doors, pick things up or manipulate them.
Although they can use speech to communicate, they may not recognize or understand the meaning of words spoken in response. We introduced the concept of 'symbiotic autonomy' to enable robots to ask for help from humans or from the Internet. Now, robots and humans in our building aid one another in overcoming the limitations of each other. CoBots escort visitors through the building or carry objects between locations, gathering useful information along the way. For example, they can generate accurate maps of spaces, showing temperature, humidity, noise and light levels, or WiFi signal strength. We help the robots to open doors, press lift buttons, pick up objects and follow dialogue by giving clarifications. There are still hurdles to overcome to enable robots and humans to co-exist safely and productively.
My team is researching how people and robots can communicate more easily through language and gestures, and how robots and people can better match their representations of objects, tasks and goals. We are also studying how robot appearance enhances interactions, in particular how indicator lights may reveal more of a robot's inner state to humans. For instance, if the robot is busy, its lights may be yellow, but when it is available they are green. Although we have a way to go, I believe that the future will be a positive one if humans and robots can help and complement each other. Journal name: Nature Volume: 521, Pages: 415–418 Date published: ( 28 May 2015) DOI: doi:10.1038/521415a. See Insight.